The interplay between Artificial Intelligence and neuroscience
The beneficial reciprocal relationship between AI and neuroscience
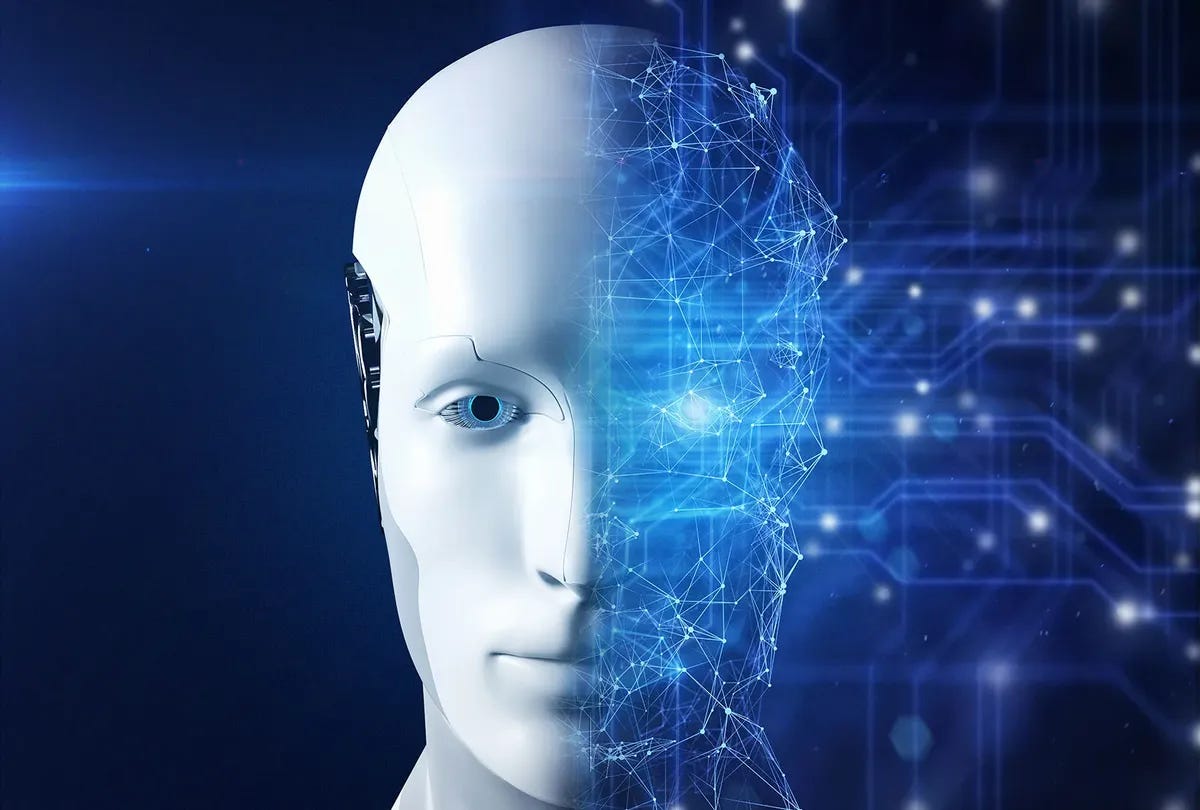
Neuroscience and artificial intelligence (AI) share a long history of collaboration. The history of this collaboration dates back several decades. In the 1950s and 1960s, researchers began using computers to simulate neural networks in the brain. This led to the development of artificial neural networks, which are now widely used in machine learning and other AI applications. In fact, the field of artificial intelligence was initially inspired by the study of the human brain. Early pioneers such as Warren McCulloch and Walter Pitts sought to create computational models of neural networks, which would eventually lead to the development of neural networks as we know them today. Today, advances in neuroscience, alongside huge leaps in computer processing power, have given rise to a new generation of neural networks inspired by the architecture of the brain.
Thus, classically, the definition of intelligence has largely been based upon the capabilities of advanced biological entities, most notably humans. Accordingly, research into artificial intelligence (AI) has primarily focused on the creation of machines that can perceive, learn, and reason, with the overarching objective of creating an artificial general intelligence (AGI) system that can emulate human intelligence, so called Turing-powerful systems. As we continue to develop more advanced AI technologies, it becomes increasingly important to understand how they interact with the human brain and how they can be used to enhance our understanding of the brain.
The beneficial relationship between AI and neuroscience
The beneficial relationship between AI and neuroscience is reciprocal, and AI is now rapidly becoming an invaluable tool in neuroscience research. AI models designed to perform intelligence-based tasks are providing novel hypotheses for how the same processes are controlled within the brain. Furthermore, advances in deep learning algorithms and the increasing processing power of computers now allow for high-throughput analysis of large-scale datasets, including that of whole-brain imaging in animals and humans, which has accelerated the progress of neuroscience research (Thomas et al., 2019, Todorov et al., 2020, Zhu et al., 2019). Deep learning models trained to decode neural imaging data can accurately make predictions of decision-making, action selection, and behavior, helping us to understand the functional role of neural activity, a key goal of cognitive neuroscience (Batty et al., 2019, Musall et al., 2019). In fact, wasn't it for these advancements, we probably wouldn't have seen companies such as Neuralink or OpenAI today. The knowledge driving such applications and innovations stems from 200 years of brain research. From the decapitation experiments that first identified specific brain regions, to the discovery of neurons as functional units of the brain. Fortunately, neuroscience now has a respectable understanding of how the brain works. However, despite all these advances, we still need to know more.
If you enjoy reading articles about the convergence of A.I. and other technologies such as nanotechnology or synthetic biology, like to be informed of the latest and breaking news around futuristic ideas and topics, Join FuturiX here. The newsletter is free for now.
How neuroscience and AI interrelate
Neuroscience has played a key role in the history of artificial intelligence. It has been an inspiration for building human-like AI. There are three ways how AI and neuroscience interrelate.
Emulating human intelligence, and
Building neural networks that mimic brain structure
Understanding how brain works using AI
1- AI systems that emulate human intelligence.
Recent AI advancements are taking the media by storm by performing impressive feats such as:
reliable object recognition that such as Tesla’s self-driving cars
playing video games to outperform the best StarCraft players
OpenAI reduces its API cost by 10x and opens it up to developers. Use cases for AI are expected to explode after this announcement. Companies like Instacart and Shopify are already jumping on it. You can read more about it here.
Elon Musk is reportedly assembling a team to build a rival to OpenAI and ChatGPT. Despite being a co-founder of OpenAI, Elon is reportedly unhappy with the political bias embedded in ChatGPT. (source)
Snapchat has entered the AI arena after announcing its own AI chatbot this week called MyAI. The feature will be available to Snapchat+ subscribers. (source)
These machines can perform tasks and solve problems better than us, achieving performance that rivals or even exceeds human capability.
Here is the list of one of the most comprehensive A.I. tools available out there, click below and access the showroom of A.I. tools I have gathered.
However impressive these may seem to be, they are only designed to excel in their intended functions. We are still decades away from building artificial human intelligence. These AI systems differ from human intelligence in crucial ways. For a machine to learn or think like a person, it would need to be able to:
explain, understand, solve real-world problems
learning-to-learn for acquiring knowledge
generalize knowledge to new tasks and situations
Therefore, researchers are now looking at building models that can make decisions like us and solve problems in the same way that humans do. Their discoveries have opened up the possibility that advances in technology might lead to building machines that are more human-like. I will go over this in my next article on FuturiX.
2- Build neural networks that mimic brain structure.
We went over the history of the influence of neuroscience and human brain on the development of AI. The idea of neurons in neural networks has similar characteristics to the biological neurons in the brain. A human brain contains about 86 billion neurons, each individually linked to other neurons. Biological neurons are cells: when one gets activated, it generates a spike and sends signals to other neurons. Like a human brain, the machine learning neural network also consists of interconnected neurons. When a neuron receives inputs, it gets activated and it sends information to other neurons.
Our brain’s allows us to learn and improve our skills. Every time we learn new things, we are creating and strengthening the connections between neurons. That’s why when we practise a task, we become better at it.
Similarly, a neural network learns when we feed it with lots of data. Each connection on the neural network is associated with a weight that dictates the importance between neurons. During the training process, the weights are tuned accordingly to strengthen or weaken the connection between neurons.
3- AI helps us to understand how our brain works.
Neuroscientists are researching how the human brain processes thoughts and how it moves our bodies. By knowing more about the brain, we are equipped to better diagnose mental diseases and enable people with disabilities to improve movement capabilities.
The advancement of artificial intelligence systems can help drive neuroscience forward and unlock the secrets of the brain. It allows neuroscientists and researchers to build better models to simulate the human brain.
Neural networks are acting as “virtual brains” that capture the representation of our brain. These virtual brains can produce patterns of neural activities that resemble the patterns recorded from the brain. These patterns allow neuroscientists to test hypotheses and observe the results from simulations before investing more resources for actual testing on animals and humans.
However, neural networks are still only an analogy of how the brain works, it models neurons as numbers in a high dimensional matrix. But in reality, our brain is a piece of sophisticated biological machinery that uses chemical and electrical activity. One area where AI and neuroscience are particularly intertwined is in neuromorphic computing. This approach seeks to create computer systems that mimic the structure and function of biological neurons. By doing so, these systems can perform tasks more efficiently than traditional computers while also being more adaptable and resilient. This interplay has various potential benefits such as:
Medical advancements: For example, AI could be used to analyze brain scans and identify abnormalities that may be missed by human doctors. This could lead to earlier detection of diseases such as Alzheimer's or Parkinson's. In addition, there are many exciting possibilities for how neuromorphic computing could be used in the future. For example, it could be used for brain-computer interfaces (BCIs), which would allow people with disabilities to control computers using their thoughts. It could also be used for autonomous vehicles and robots, which would require real-time processing capabilities.
Prosthetics: By combining AI with brain-computer interfaces, it may be possible for amputees to control prosthetic limbs with their thoughts or developing prosthetics that respond more naturally to a user's movements.
Creating more personalized learning experiences for students based on their individual brain activity.
Creating more intelligent robots that can navigate complex environments with ease.
The concept of neuromorphic computing is based on the idea that the human brain is incredibly complex and efficient. It is capable of processing vast amounts of information in parallel, using a network of neurons that communicate with each other through electrical signals. By modeling computer systems on this network, researchers hope to create machines that can perform tasks more efficiently than traditional computers.
Neuromorphic computing have key advantages compared to common computers or computing in general (I will post the difference with Quantum Computing for those who might be confused) that I have presented below:
Ability to process data in real-time. This means that it can be used for applications such as image recognition, speech recognition, and natural language processing.
Potential to be much more energy-efficient than traditional computing systems, which could have significant implications for reducing carbon emissions.
Robustness: Neuromorphic computing systems are designed to be resilient and flexible, enabling them to keep running in the face of failures or defects.
High Accuracy: Pattern recognition and classification tasks may be completed by neuromorphic computing systems with high degrees of accuracy.
Learning Capability: Due to their capacity to draw on their existing knowledge and adjust to new input, neuromorphic computing systems are perfect for use in applications that need machine learning.
There are also some challenges that need to be overcome before neuromorphic computing becomes mainstream:
Developing algorithms that can effectively utilize the capabilities of these systems.
Developing hardware that can scale up to handle large-scale applications.
Complexity: Due to their complexity and potential difficulty in design and implementation, neuromorphic computing systems need for specific knowledge and experience.
Cost: The development and implementation of neuromorphic computing systems can be expensive, which limits their usability by small organizations and individuals.
Limited Availability: It is challenging for companies and people to obtain and use neuromorphic computing systems since they are not generally available.
Limited Processing Power: Neuromorphic computing systems may have less processing power than conventional computing systems, which makes them less suited for some applications that demand high performance.
Security Issues: Users must take precautions to safeguard their data and information since neuromorphic computing systems may be susceptible to security risks.
Examples of neuromorphic computing being used in real-world applications already exist. For example, IBM has developed a neuromorphic chip called TrueNorth, which is designed for use in cognitive computing applications. Another example is Qualcomm's Zeroth platform, which uses neuromorphic techniques for image recognition and other tasks, and Intel's Loihi 2 which is a New Generation of Neuromorphic Computing.
Best time to Start a Newsletter especially if you're interested in A.I. and futuristic technologies!
This section, I picked up from Michael Spencer! He was the sole person who inspired me to start my own newsletter. So, I'm also inviting you to join. If you want to write a Newsletter, I think you should just do it! In 2023, Substack is the best discovery, growth and retention platform to gain new audiences and build valuable niche communities. There are also other platforms such as Beehiive. You can follow its founder Tyler Denk 🐝 if you'd like.
I’ll be thrilled if you started a Newsletter today, since we’re all learning together right?
How Will Broad AI Affect Humans?
Now to the topic that keeps Elon Musk awake at night, or at least lingers in the background, how will AI take over our lives and will we survive the singularity?
It's kind of evident that AI will take increasing control over our lives. Not in a Terminator-style theme hopefully, but a fast-paced infusion of technological advancements that can benefit our future on this green blue earth! Soon, AI will dramatically change our stock markets, our hospitals, and our infrastructure, but we're all hoping that it won't take control over us and our lives. That is probable, unless we merge with AI. Then we can take an active role in its evolution, ensuring that the resulting superintelligence sees humans as a symbiotic species—and inherently indispensable. Well, this is how Elon Musk thinks we can escape Terminator's starting to kill us all.
"We can actually go along for the ride... and we can effectively have the option of merging with AI." - Elon Musk
Such a proposition is arguably more terrifying than having a Neuralink installed. No-one truly knows what will motivate AI, whether it will engage in any kind of morality, or whether it will take on an agenda so bizarre we won't even be able to rationalize it. We must, at least, have a seat at the table.
No discussion of AI would be complete for me without mentioning science fiction movies like The Matrix. An interesting application of neuromorphic computing is depicted in these movies where machines become self-aware and take over humanity or where humans merge with machines creating cyborgs with enhanced abilities beyond human limitations.
Buckle up, Superintelligence is coming!
Recommendation:
I promised to recommend and introduce similar newsletters or podcasts on AI on every episode. So, if you enjoy articles about A.I., you can also join:
Artificial Intelligence Survey 🤖🏦🧭
Bite size curation of links to A.I. News, funding and trending topics from around the web.
References:
2- The Fascinating Relationship between AI and Neuroscience
3- Elon and Snapchat enter the AI wars